Revolutionize your paper manufacturing with AI-driven optimization. Enhance efficiency, cut costs, and achieve autonomous operations like industry leaders Arjowiggins and Oji Paper. Embrace the future of smarter production!
Learn more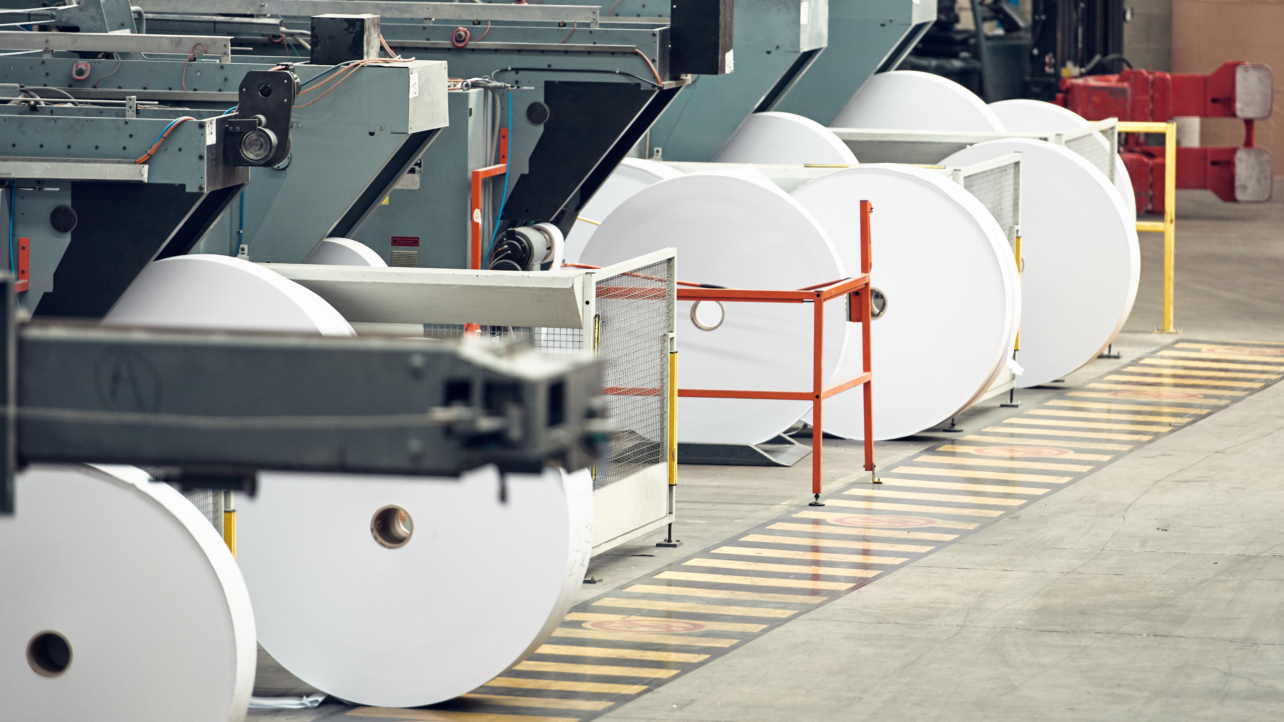
This white paper provides strategies to improve day-to-day manufacturing performance and long-term benefits with proven IIoT use cases.
Repeating golden runs or batches in Industry is a priority for many manufacturers. Many industries are still facing tumultuous supply and demand prices and availability, making each run of utmost importance.
Golden batches and/or runs aim to give manufacturers the ability to repeatedly produce an accurate product. They encapsulate the ideal production parameters necessary for equipment, materials, process, and all other aspects of production to flow together simultaneously.
While there are many upsides to golden batches, if it were easy to master, all products would undergo the same process. Yet we know that variability is inevitable when making complex products. To not only master a golden batch by product, but by an entire product line or even multiple lines takes time, expertise, and of course, the right data and teams to execute.
According to McKinsey’s research on process modeling, manufacturers implementing advanced tech in manufacturing see tremendous ROI. In fact, they report a reduction in deviations of more than 30 percent and a reduction in the overall cost of quality by 10 to 15 percent.
Even though manufacturers know more about their processes than ever before, it can be too complex and time-consuming to constantly be pulled away from production to uncover better, more efficient processes. But doing so might yield more than you might think.
If this rationale alone does have you thinking of a golden future, it’s worth reading on to see why other leading manufacturers have embraced Golden Runs.
“What would have taken hours of work going through 4000 different data tags that we use on our machine, we’re able to do in just a few clicks,”
Tyler Shirley, Asset Development, Electrical Engineer at Kimberly-Clark.
Consistent and reliable data means that you have a way to utilize your historical and live data. You need your historical data to understand the settings that lead to golden runs, and you need an ongoing stream of live data to adjust and adapt accordingly.
If you have historical data, you’ve likely been able to leverage AI, Machine Learning, or your data scientist team to identify your centerline and at least have stabilized your process. In turn, the next logical step is to focus on repeating and further refining your golden runs. To do so, you may want to look at Digital Twins, which can help you to provide a continuous data model that provides an as-built snapshot of everything you produce.
Data may be an asset for many manufacturing companies, yet having data isn’t enough. Say, for example, you have access to understanding the average boiler temperature on one of your lines. Sure, that’s interesting information to have, but what can you do with the average? It doesn’t tell you what to change, it simply tells you what the temperature was on average over a period of time.
To repeat golden batches, you need to identify the optimal set points across your product lines to really ensure that you’re making the best use of your data. But to do so, you need to have the right data, which can be a longer process.
Digital Twins can help you to identify how to manufacture your product with a high yield and low cost. Braincube-powered Digital Twins equip teams with a dynamic digital replica of how a product was made. In turn, you can use the Digital Twin database in third-party systems, Braincube applications, or other custom-built models.
For example, Kimberly-Clark uses Python to build predictive models. This helps them to understand how to optimize basis weight and other standards to generate their golden batches. However, we both know that models are only as good as their data.
To ensure the right data was going to be used, they leveraged Braincube’s Advanced Analysis app to validate the model. The AI identified that there were surprising pieces of data in the original model. These data points were actually incorrect! Wrong tags had mischaracterized the data, showing inconsistencies that did not exist.
“What would have taken hours of work going through 4000 different data tags that we use on our machine, we’re able to do in just a few clicks,” said Tyler Shirley, Asset Development, Electrical Engineer at Kimberly-Clark.
The right data and tools for analysis can save companies time, and resources, and reduce overall production costs. Oftentimes, AI can even uncover unexpected optimization opportunities.
You can’t, however, just dive into golden batches. Typically, they require a large amount of historical data. You must understand different runs that have been both successful and unsuccessful. Ones that hit your quality mark and took fewer resources to produce.
This is often done by integrating some type of Advanced AI, such as Braincube’s CrossRank or Machine Learning to detect patterns that lead to positive results. Tools like this are able to identify your optimal runs, continuously adapt to changing conditions, and offer tools to send changes to the shop floor in a single platform. By understanding historical performance, you can start to uncover the ideal settings for whichever product you’re producing.
Interestingly enough, though, sometimes what you find is not what you might expect. For example, a paper manufacturer we work with saved the cost of purchasing new equipment because they were able to make some adjustments identified by Braincube’s analysis tool that allowed them to maintain the same quality with fewer resources.
Oftentimes AI will help you to identify patterns, but it does not often also tell you the prescriptive operating settings for repeating your success. We all know that manufacturers can’t afford to take risks–if they manipulate something upstream, they need to understand the implications on final quality, machine wear and tear, and other breakdowns in production.
For example, just because you want to reduce your energy consumption, doesn’t mean that you can isolate that KPI. You must understand how to reduce energy without sacrificing other factors like quality, speed, or throughput.
You must consider the theory of constraints and the influences you may have downstream if you’re not cohesive in your approach. Oftentimes manufacturers may be too focused on a handful of KPIs causing overall performance to drift.
On the other hand, you must know what you want to achieve with golden batches. Are you willing to burn more energy for better quality? Is it necessary to be in lights out mode, getting every possible product out the door, knowing some products are better than others? Is your process agile enough that as energy sources fluctuate, you have a plan to pivot?
A global food and beverage manufacturer and Braincube customer wanted to reduce their gas consumption and still maintain their quality. With the rising cost of gas, they wanted to find more sustainable options, including steam generation with biomass boilers instead of reliance on gas. As a major name brand, this company couldn’t afford to sacrifice quality.
They felt that if they could replicate their golden batches by focusing on their sustainability KPIs, they would save substantial money and keep teams motivated. With more than 1300 variables from the coffee treatment process and biomass boiler phase, there was a lot of data to dissect. They specifically wanted to ensure that their biomass boiler, which produces steam to extract water-soluble coffee material, was a focus as it consistently demonstrated a lower than their machine average OEE score.
Being able to dissect their process helps them understand inefficiencies in their biomass operation. They used AI from Braincube to determine that they could lower the water content in their product with AI-optimized settings to regulate the CO2 level. Doing so helped them to reduce their gas consumption by 21,600 tons. They also reduced their annual CO2 emissions by 4,200 tons.
Making reductions and precise changes like this, help build the data needed for a golden batch. Now that they have golden batches down, they are able to compare different active rules, view their average application rate, results & trends, and uncover optimizations that lead to multi-million dollar savings. Because Braincube is designed to close the production loop, the team can send settings directly to frontline teams with just a few clicks.
This continuously connected process is why many customers stay with Braincube.
A long-time customer in paper manufacturing wanted Braincube to help them identify how to repeat golden batches. They use this information to measure against their process stability goals. If teams can achieve golden batches time and time again, they are consistently implementing the right production parameters for a given product.
Braincube’s tools make it easier for teams to look at prior runs, choose the runs with the best conditions, and provide operators with guidance about how to set up the next run. For example, using Studio, a central portal leading to endless industrial use cases, allows individuals to benefit from a highly customizable experience.
For example, teams or individuals can select the grade and speed range they’d like to view and pull the golden batch of a given product. Then, the app dashboard automatically populates with the running conditions of key input variables from the most recent “golden batch.” In this sense, the dashboard recreates a rule/production standard in real-time based on the most current data, giving operators guidance as they set up for the next grade run.
However, it doesn’t mean that teams can repeatedly run the same parameters every time and expect the same results: like any manufacturer, this customer needs to continuously adjust for set-point drift, grade changes, asset performance, and other variables.
“If you just go back to the last settings you ran, after about six or seven runs, you realize you’ve drifted off into some poor setting,” said this customer. “No one really notices it happening, but it happens. With Braincube, teams can evaluate what happened and ask themselves, ‘How do we run this next grade best?’”
By pulling the most recent “golden run” settings into the dashboard, this company’s employees can account for some process drift by not relying on what worked many months or years ago. They are continuously using the most up-to-date settings that lead to good performance.
The paper industry has tight margins, and with the rising cost of oil to the challenge of sourcing raw materials, many costs are continuing to increase in cost and decrease in availability. This is where recipe optimization and golden batches really improve companies’ competitiveness.
Understanding how much filler can go into a product, while maintaining the quality, or identifying the lowest heat settings necessary to melt glass, can help save money on materials.
“In the past, figuring out how to upgrade a production line without AI was a real puzzle. The data that needed to be processed was highly complex,” said the Manufacturing Director at Saint-Gobain-Weber. “There were multiple factors that had to be taken into account in real time. Plus, we didn’t take into account the time required to analyze data and make decisions.”
Ok, maybe we should have led with this. But we had to save some food for thought at the end. While many of our customers use the Braincube product suite to manage golden runs and do it well, we encourage you to consider your own definition of a golden run.
For example, much of the time, companies try to repeat runs based on their historical runs, and of course, there is a time and place for this. However, with our CrossRank AI, what we’re trying to help you identify isn’t just repeating a run, but rather understanding what can be consistently achieved with different dimensions of a golden batch.
When you run an Advanced Analysis within the Braincube platform, you’re actually defining the outcomes you want to achieve, then the algorithm will prescribe how to achieve them. For example, maybe energy savings is what you need more than anything, so you want to look at a golden batch using alternative energy sources. Or perhaps you want to use less costly material and add the highest volume of filler you can use before impacting quality. Regardless of need, one of the key differences in how Braincube approaches golden runs is this: we add context by cross referencing past runs to recommend the right settings based on your end goal: volume, energy, quality, etc.
While it’s true that you need a large amount of data to propose a golden run, tools like Braincube are here to help. From data visualization and storytelling to robust self-service analytics, our crawl, walk, run approach to digital transformation will help you not only reach your goals but actually exceed them.
With the right tools, data, and strategy results that rely on golden batches are nothing short of transformational.
Implementing tools like Braincube provides customers with the tools and resources that enable them to think creatively and critically about what happened during production and why. Engineers and operators still have autonomy about what parameters to implement, but they are making better data-driven decisions because they can quickly and easily access data filtered and analyzed in real-time to provide the most optimal recommendations based on recent success.
The IIoT Platform is your foundational infrastructure with robust security, collaboration, and administration settings. Discover instant and long-term value from a centralized data hub with business intelligence apps and Digital Twins.
Edge analytics is the process of adding intelligence—such as visualization or alerting—to Edge data. Get answers to your top questions about Edge analytics in this introductory article, including how it works and the value it brings your organization.
In this case study, learn how leading manufacturer of paper and personal care products, Kimberly-Clark was able to leverage its Digital Twin data to build a predictive data model to optimize processes with high accuracy.