Revolutionize your paper manufacturing with AI-driven optimization. Enhance efficiency, cut costs, and achieve autonomous operations like industry leaders Arjowiggins and Oji Paper. Embrace the future of smarter production!
Learn more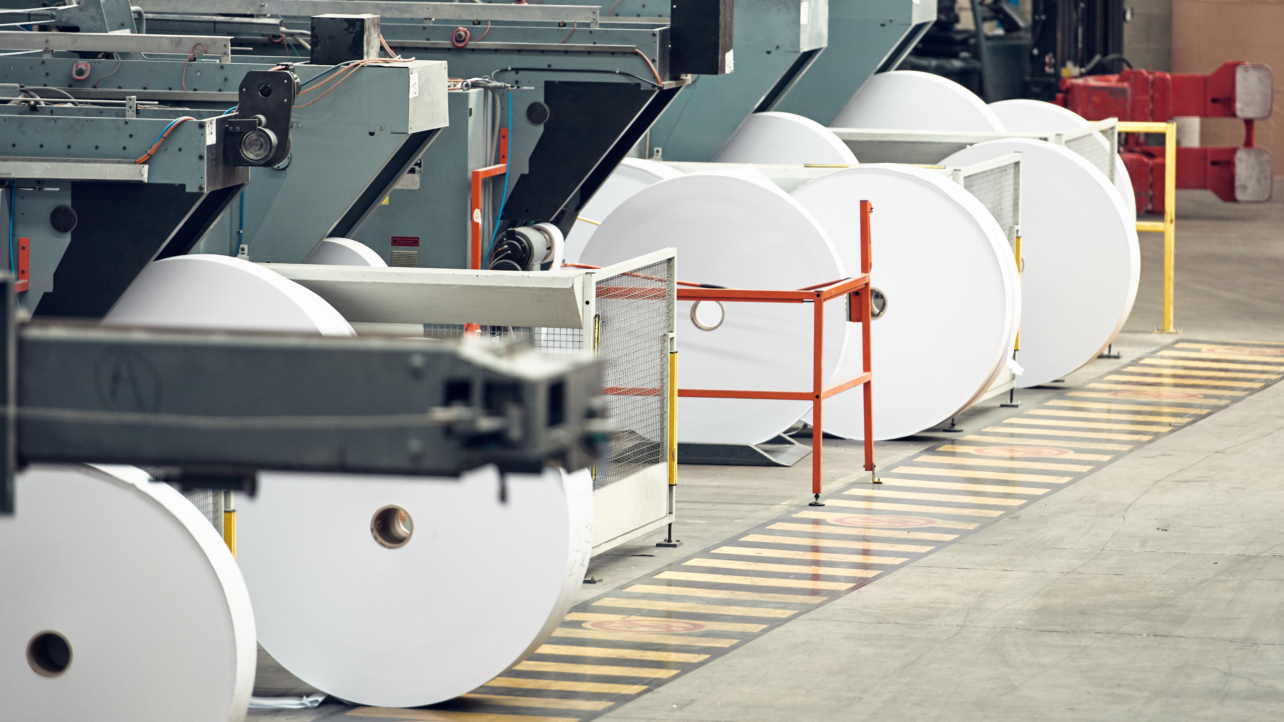
Download this article as a PDF to share, read, or reference later.
During our recent Braincube customer summit, manufacturing leaders shared their lessons learned about the industrial technology landscape. A key recommendation, which has generated millions of dollars in savings at their own companies, focused on specialization. Finding technology that does exactly what you need to achieve business goals is more valuable than trying to finagle a generic solution.
With Generative AI, closed-loop control, and other next-generation technologies, how can you align a roadmap to support your business today, while growing into your future state? Like any major shift, success includes orchestrating an organizational shift to support a digital mindset that grows profits, supports your people, and reduces environmental impact.
Below are lessons we’ve learned as a leading digital manufacturing platform over the last 15 years.
With a massive staffing shortage in industry, finding efficiency from the shop floor to the board room is critical. To address this challenge, should you lead by supporting technology or by business outcomes? While you don’t always have to choose, below is one of our customer’s “lessons learned.”
Wearables and frontline enablement solutions strive to make critical teams more efficient by connecting workers (literally) to technology. Many wearables or “mobile-first” frontline solutions require 5G to be most effective.
This means most manufacturers must invest significantly to upgrade their architecture before deriving the full value of these solutions. The reality is that most plants still operate with legacy equipment, have limited bandwidth, and struggle to justify new equipment expenses and infrastructure.
Instead of leaping full force into 5G, consider where 5G is truly necessary. If it’s for making real-time performance data accessible to the shop floor, going “all in” on mobile-first and wearables will likely fall short of your expectations. If you want 5G for interactive maintenance, find the best vendor.
5G will play a critical role in the future of manufacturing. However, based on this leading manufacturer’s “lesson learned” reminds us to meet our plants where they are with business-led outcomes—then follow up by bringing in supporting tech.
In other words, it can be more effective (both financially and from a tech-adoptive standpoint) to find solutions that match your digital maturity today and set you up for the future. This means your digitally mature plants can be your incubators. Other facilities can use existing tools that are less costly and more reliable for delivering the correct information to the right place at the right time—for instance, web-based solutions that leverage cloud and edge data.
A digital transformation expert was tasked with bringing faster, more actionable data to production teams to improve performance. The corporate management team recommended a mobile-first strategy. They would fund a plant with the hardware and software needed to drive frontline enablement.
Companies like Redzone specialize in mobile-first solutions. “Using real-time, social mobile communication, Redzone software connects and unifies team members across departments to create ‘one team’ working with purpose,” according to the company’s website. Redzone describes itself as the “#1 connected worker solution.”
As mentioned, mobile-first approaches typically require infrastructure upgrades, which come with their own set of challenges. In fact, their solution page states, “There’s even a “bat-phone” for when things go wrong”—implying that reliable support is a must.
As this manufacturer got deeper into the scope of their 5G project, they realized that their facility could not support the 5G bandwidth required. A mobile-first strategy would simultaneously make them hardware-dependent and increase their security threats. The manufacturing company pulled out from their mobile-first initiative and went back to the drawing board.
Ultimately, they didn’t need a mobile-first strategy. The business need was better shop floor visibility. They wanted to make data-driven decisions instead of working on intuition and habits.
The company installed large monitors that display real-time performance and provide frontline teams with the data they need to improve production. This device-agnostic solution displays Braincube’s Studio, a web-native interface. Studio keeps the company’s teams in the know with key indicators like line speed, slices per minute, and asset settings.
For just a few hundred dollars, the company now leverages monitors to improve its production outcomes.
Another Braincube customer took a similar approach. They even gamified the shop floor. By turning production outcomes into a game, the company drives friendly competition between shifts, increases collaboration across departments, and drives great profits for the company.
Wearables and 5G are not going away. However, they aren’t the right solution for every company today. In the competitive world of manufacturing, finding tools that can serve your needs today (and, ideally, in the future) is critical.
Ultimately, the choice between a web browser or a native app depends on factors like the target audience, required features, and development resources. Braincube’s hybrid approach uses web-based technologies that can be displayed on large or mobile devices in addition to computers. For many companies, this provides the ideal balance between cross-platform compatibility and cost-effective development.
International Paper utilizes Braincube’s data visualization tools to reduce variability, improve compliance, and adapt production to real-time conditions.
As the manufacturing labor shortage continues, many companies are using automation to shrink the skills gap with greater operational efficiency. Because this shortage impacts both technical and non-technical alike, IT/OT alignment is critical to success.
Another “lesson learned” from a Braincube customer focused on how data should be used holistically across the organization without requiring advanced technical expertise. How data is used and shared across the organization should matter to all employees.
Yes, IT should drive the data strategy, but they should not be on the hook for continuous execution. If leaders are to be effective, they cannot spend 2-3 hours building reports and cobbling together different systems and formats. Similarly, production will also be more effective with live data.
Many industrial technologies continue to focus on expert users. This includes IT, data science teams (aka “technical teams”), and engineers who understand processes. It may also include maintenance teams looking for advanced maintenance software solutions or even an ERP for financial reporting.
Even though one vendor cannot do all things well, there are strategies to align teams on common business goals. This ensures that all users are given the right information, in the right format, at the right time.
When it comes to data consumption and usage across the organization, giving teams “more” data doesn’t necessarily mean employees are gaining more value from that data. Bringing in holistic solutions that provide value across multiple departments can also be highly successful.
To be clear, experts do need tools that are purpose-built for highly technical objectives. However, these experts must speak a common language with other departments. When vendors offer per-license pricing, it can isolate departments by further siloing data. This typically widens the data-knowledge gap that exists at so many manufacturing companies rather than closing it.
—Executive at a leading paper manufacturing company
In other words, if you only put advanced tools in the hands of your data experts, it’s unlikely you’ll get the results you’re looking for. Most companies find more success when they democratize data and provide all employees with tools to understand and derive value from data.
For example, Seeq, an ML and AI platform, says that they will “help you find solutions that are relevant to your role in your industry.” Their different packages are geared towards roles. However, it’s unclear how these role-based solutions align across the organization.
G2 explains that Seeq offers licenses by enterprise (more than 35 users) or teams (10-35 users). While these licenses are further customized by role-based offers, they also create a divide within the company.
A leading executive at a paper and pulp producer shared that his company was moving away from paying for software solutions on a per-user basis. Every time an employee was hired or someone resigned, the company had to manage another license set-up or transfer. This administrative headache caused more struggles than it was worth, taking valuable time away from IT teams.
“One of the advantages of using Braincube is the unlimited user subscription model,” said an executive from a leading paper manufacturing company. “This subscription model encourages our employees to use advanced tools and supports our enterprise needs. Teams are encouraged to collaborate across sites and departments, which is something per-license vendors miss.”
Everyone is using Braincube, yet in an integrated and personalized way.
Amidst advancing technology, manufacturers maintain that data quality is critical to successful implementation. Connectivity is usually manageable by using connectors. Public clouds, such as AWS, Azure, and GCP, all flourish in data extraction and storage.
Yet the needs of today’s manufacturers go beyond storing data. Data must be verified and formatted so that non-technical teams trust the data and, therefore, are willing to use it. In a world where many manufacturers are skeptical of AI’s validity (and other generative technologies), getting it right the first time can be critical.
At a recent manufacturing conference, two manufacturing executives were debating generative AI and predictive models. Could ChatGPT build an effective predictive industrial model? They determined that this may be possible, but that they wouldn’t trust it.
Quality data is a critical sticking point for culture change.
For example, predictive models run by non-process experts can help with quality control, predictive maintenance, yield predictions, and other goals. They rely on data science, machine learning, and statistical models to answer questions like, “What is likely to happen?”
However, these models can fall into a “data discovery” that is unrealistic. Predicting that a boiler should run at an impossibly high temperature or that a belt should speed up to a known breaking point are simplified versions of poor data quality.
A lack of process knowledge can often be mitigated with solutions designed specifically for process experts. This allows data scientists to run AI/ML models that drive progress in tandem with process teams.
For example, RapidMiner, Seeq, Dataiku, and Braincube equip non-technical teams with technologies that develop automated predictive models leveraging automated AI and ML. Solutions like this help improve data validation. This allows process experts to optimize processes instead of data mining.
Unlike most solutions, Braincube’s prescriptive models go beyond recommendations. Instead, Braincube models tell you how to optimize outcomes based on the goal you’re trying to solve.
Braincube’s Advanced Analysis App uses AI to provide specific set points to achieve your desired outcomes.
For example, Braincube’s solutions can help teams answer specific questions, such as “What temperature should I operate my boiler to guarantee on-spec products with the lowest possible steam consumption?” On the other hand, prescriptive models can only answer questions like, “What should be done to achieve a desired outcome?” instead of telling you what is likely to happen.
Using AI, Braincube crunches historical data to provide you with the exact criteria (such as set points, speed, temperature, etc.) you should apply for your ideal outcome (e.g. lowest energy, lowest cost to produce, quickest cycle time, etc.).
Prescriptive models also require up-to-date information to ensure that the prescriptions are relevant. This data can be automated to update in real-time with digital twins.
Predictions use data to hypothesize what is likely to happen. It’s commonly believed that if a model can predict it, then we also can avoid it!
But this relies on many things to go right.
First, you must have enough data to accurately predict what you need to know. This can be effective for forecasting or predictive maintenance. However, when it comes to complex manufacturing processes, predictive models are limited.
Predictive models provide you with more data, but they still rely on a person’s interpretation of that data. This is a common challenge with predictive models. Just because you know something may happen, you don’t know why it will happen or exactly how to prevent it—unless it’s something you’ve learned over time…and even then, you’re relying on experience, not data.
When it comes to digital twin technologies (which are typically used to feed these models), companies like TwinThread rely on time-series data to “shift from reacting to challenges and disruptions, to actively anticipating and avoiding them.”
Time series data is valuable for analyzing trends, patterns, and making predictions over time. However, this kind of data doesn’t connect with how a product was made. Time series data limits your view to a 2D trend line. Solutions like Braincube enable teams to view data from multiple angles: two, three, four, or five dimensions all converging for a full 360-degree view into production.
In summary, predictive models are focused on forecasting future events. Prescriptive models also predict outcomes but also recommend actions to achieve desired results.
Both types of models play crucial roles in leveraging data for informed decision-making and process optimization in manufacturing. Many manufacturing scenarios benefit from a combination of predictive and prescriptive analytics to achieve a holistic and proactive approach to operations.
When you have millions of dollars on the line, you need to place the right bets. Sure, you can maintain linear growth, moving slowly and steadily, by doing things the way they’ve always been done.
But there’s another choice: you can adopt a digital mindset and revolutionize your thinking.
Start by ensuring that you understand the needs of stakeholders that will use the tool. Then, find solutions that specialize in your business objectives (rather than the technology itself).
With the right digital mindset approach, you can break out of traditional methods and gain massive achievements.
Download this article as a PDF to share, read, or reference later.
International Paper utilizes Braincube’s data visualization tools to reduce variability, improve compliance, and adapt production to real-time conditions.
Selecting an IIoT platform vendor doesn’t have to be a daunting process. With over 15 years of experience working with manufacturers, here are our top tips for making the most of your IIoT demo experience.
In this webinar from the 2022 ARC Advisory Group Conference, Kimberly-Clark’s team explains how they implemented closed-loop production processes with Braincube.