Revolutionize your paper manufacturing with AI-driven optimization. Enhance efficiency, cut costs, and achieve autonomous operations like industry leaders Arjowiggins and Oji Paper. Embrace the future of smarter production!
Learn more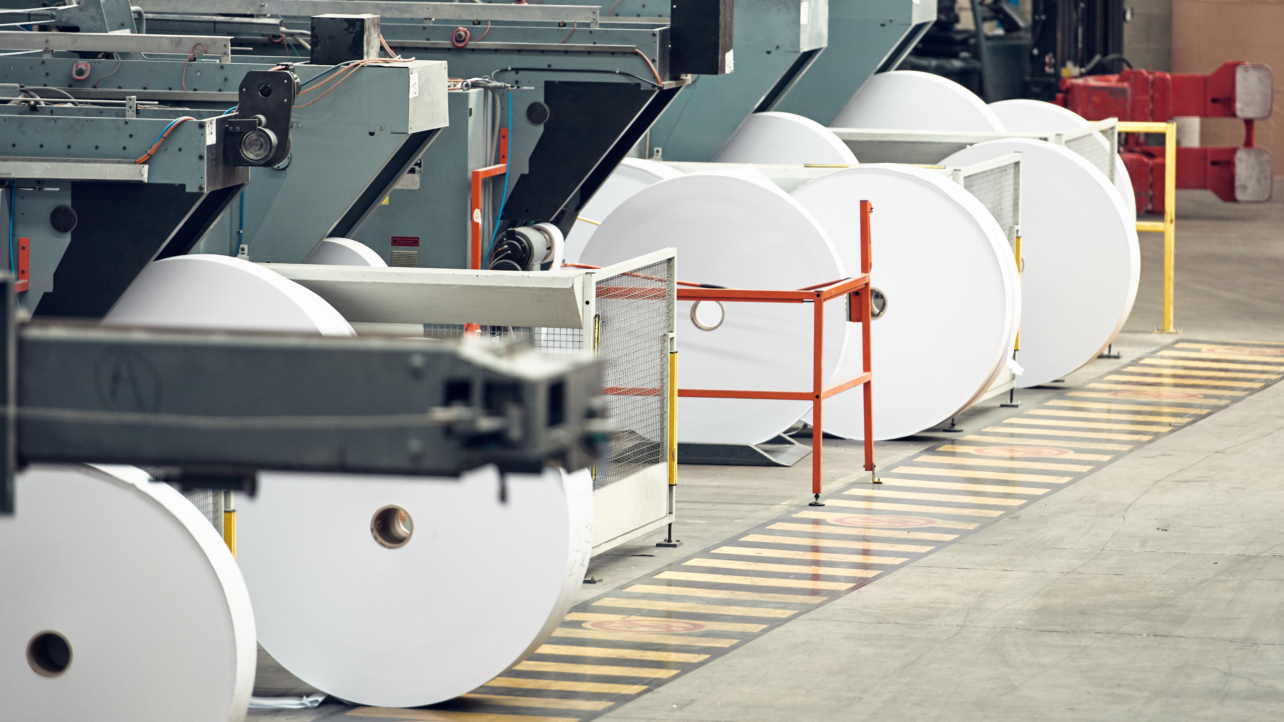
June 4, 2020
Under the generic term “Artificial Intelligence” hides a reality which is now quite simple to grasp: AI, in the general sense, is an artificial superpower capable of thinking with supposedly more intelligence than humans possess. (I recommend the latest piece by Luc Julia, the creator of Apple’s Siri. His work accurately explains the reasoning for the current tricks of AI.)
Today’s technology is a myriad of specialized solutions to assist, augment, or replace humans in certain intellectual tasks. In many ways, AI is no different than the physical robots programmed to complete specific tasks with greater speed, precision, or repetition, or allow humans to avoid dangerous environments.
Specialized AIs are virtual robots, intended to make better decisions than humans.
The technology that exists today will continue growing at a faster and faster rate. Our professional, social, and cultural environment is becoming increasingly digital. Many new applications will emerge. The goal of these apps will be to decide “the truth” in their given tasks. If an application has a potential ethical impact, its legitimacy will likely depend on the app’s intrinsic integrity: but who will guarantee or determine these ethics?
I am the President of Braincube, a company that develops solutions dedicated to optimizing automated production processes for manufacturers. In order to “learn” historical production conditions and propose actions for adjusting machine control parameters, we use probability-based calculation technology. These solutions utilize Artificial Intelligence and Machine Learning.
Every day, our products help production teams improve. We answer some of their questions or offer them prescriptions at the right time. But how legitimate are our solutions? In what way can we ensure the suggested parameter modifications that we propose are the “best”?
Braincube’s AI recommendations rely on two factors: the quality of historical data and the mathematical accuracy of our algorithms. The factories that we work with provide us with large amounts of data which, while imperfect, offers a fairly descriptive output of their production conditions.
We have built an AI algorithm that trains itself through Machine Learning, starting with small databases and increasing in scope. While this type of tool can be imprecise with smaller data sets, large data sets make this tool more reliable than other options. The algorithms that we use are based on high-quality, probabilistic, optimal search formulas—grounded in a mathematical model that rapidly crunches Big Data. Essentially, we are able to “mathematically justify” our results to provide customers with the best possible outcomes from the quality of their available data.
We offer a proven, reliable solution with over a decade of customer use. Other companies are developing applications with the same research approach, but I believe that legitimacy trumps accuracy.
I no longer speak of truth, but of accuracy.
In contrast, many current applications on the market use other technology. The most discussed type of AI focuses on a certain kind of learning technology: neural networks. Since the rediscovery of buried theories dating back to the 1980s, many researchers and engineers have embarked on the advanced use of the neural network theory in the hopes of working miracles. Looking at their work today, this technology is far from its expected miracles. Instead, neural networks have led to very poor results, such as low accuracy rates for pharaonic energy consumption.
That said, daily applications use trained neural networks. This presents a sensitive subject: what data is used for training these neural networks? The answer certainly depends on the context, but most of the data used to train these neural networks are qualified by people, not automated digital systems.
Sometimes these data selectors are experts (like radiologists), but often these people are anonymous and hired for a specific project. This means we are using inefficient learning systems—which are subject to a high probability of errors—which are trained using data with an uncontrollable quality level.
How are we to trust such solutions? Most of them have no legitimacy, and their design or learning biases make them dangerous or useless.
Don’t be fooled by companies with self-serving interests or flashy bells and whistles. Either a system provides real, estimable accuracy and truly helps with your tasks, or it is unacceptable to use because it lacks quality, reliability, and accuracy.
Every other product we use or consume has a minimum level of certification. The industrial food we eat is certified and controlled. Who would want to fly in unreliable planes? The services we use are often cursed and certified. We must consider AI a tool and, therefore, determine how to legitimize it via standardized certifications.
It is imperative that we offer users a way to identify the accuracy quality of these tools by establishing an AI assessment and certification system. Companies go to great lengths to protect their data. Now the time has come to protect ourselves from using data poorly or inaccurately. Without legitimacy, AI is a real danger.